Written by Manouchehr Rafie, Ph.D. | CEO | Gryfalcon
Autonomous Vehicles and AI Chip Innovation
Convergence of Edge Computing, Machine Vision and 5G-Connected Vehicles
Today’s societies are becoming ever more multimedia-centric, data-dependent, and automated. Autonomous systems are hitting our roads, oceans, and air space. Automation, analysis, and intelligence are moving beyond humans to “machine-specific” applications. Computer vision and video for machines will play a significant role in our future digital world. Millions of smart sensors will be embedded into cars, smart cities, smart homes, and warehouses using artificial intelligence. In addition, 5G technology will be the data highways in a fully connected intelligent world, promising to connect everything from people to machines and even robotic agents – the demands will be daunting.
The automotive industry has been a major economic sector for over a century and it is heading towards autonomous and connected vehicles. Vehicles are becoming ever more intelligent and less reliant on human operation. Vehicle to vehicle (V2V) and connected vehicle to everything (V2X), where information from sensors and other sources travels via high-bandwidth, low-latency, and high-reliability links, are paving the way to fully autonomous driving. The main compelling factor behind autonomous driving is the reduction of fatalities and accidents. Realizing that more than 90% of all car accidents are caused by human failures, self-driving cars will play a crucial role in accomplishing the ambitious vision of “zero accidents”, “zero emissions”, and “zero congestion” of the automotive industry.
The only obstacle is vehicles must possess the ability to see, think, learn and navigate a broad range of driving scenarios.
The market for automotive AI hardware, software, and services will reach $26.5 billion by 2025, up from $1.2 billion in 2017, according to a recent forecast from Tractica. This includes machine learning, deep learning, NLP, computer vision, machine reasoning, and strong AI. Fully autonomous cars could represent up to 15% of passenger vehicles sold worldwide by 2030, with that number rising to 80% by 2040, depending on factors such as regulatory challenges, consumer acceptance, and safety records, according to a McKinsey report. Autonomous driving is currently a relatively nascent market, and many of the system’s benefits will not be fully realized until the market expands.
AI-Defined Vehicles
The fully autonomous driving experience is enabled by a complex network of sensors and cameras that recreate the external environment for the machines. Autonomous vehicles process the information collected by cameras, LiDAR, radar, and ultrasonic sensors to tell the car about its distance to surrounding objects, curbs, lane markings, visual information of traffic signals, and pedestrians.
Meanwhile, we are witnessing the growing intelligence of vehicles and mobile edge computing with recent advancements in embedded systems, navigation, sensors, visual data, and big data analytics. It started with Advanced Driver Assistance Systems (ADAS), including emergency braking, backup cameras, adaptive cruise control, and self-parking systems.
Fully autonomous vehicles (AV) are gradually expected to come to fruition following the introduction of the six levels of autonomy defined by the Society of Automotive Engineers (SAE). These levels range from no automation, conditional automation (human in the loop) to fully automated cars. With increasing levels of automation, the vehicle will take over more functions from the driver. ADAS mainly belongs to Level 1 and Level 2 of automation. Automotive manufacturers and technology companies, such as Waymo, Uber, Tesla, and a number of tier-1 automakers, are investing heavily in higher levels of driving automation.
With the rapid growth of innovations in AI technology, there is a broader acceptance of Level 4 solutions, targeting vehicles that mostly operate under highway conditions.
Although the barrier between Levels 3 and Level 4 is mainly regulatory at this time, the leap is much greater between Levels 4 and 5. The latter requires achieving the technological capability to navigate complex routes and unforeseen circumstances that currently necessitate human intelligence and oversight.
As the automation levels increase, there will be a need for more sensors, processing power, memory, efficient power consumption, and networking connectivity bandwidth management.
AI Chip Challenges
Demand for deep-learning accelerator (DLA) chips continues to be strong in applications deployed throughout the industry from data centers to self-driving cars to edge devices and embedded (IoT) systems. Cutting-edge chips are necessary for AI because it requires a specific architecture that is more appropriate for AI application processing. The desire of the AI chip to process at the edge is strong for a variety of reasons: Locality, low latency, privacy/security, mobility support, and power consumption restriction.
The trend is to use Tensor architecture as opposed to Linear or Vector processing that is typically used in CPU, DPU or GPU, respectively. While the movement of data into and out of AI chips (data bandwidth) is a big challenge, making sure that linear algebra and matrix operations can be done efficiently at the highest level of throughput with the lowest amount of energy is other common challenge. Cutting-edge chips or dedicated co-processors are becoming the mainstream for on-device, edge, and even cloud AI processing.
Autonomous vehicle technology is difficult because it requires processing massive data captured by the sensors (camera, LiDAR, Radar, and Ultrasound). And it has to provide real-time feedback, such as traffic conditions, events, weather conditions, road signs, traffic signals and others. This requires high trillions of operations per second (TOPS) to process multiple challenging tasks (e.g., object extraction, detection, segmentation, tracking, and more) simultaneously. It also consumes high power consumption depending on the operation. Lastly, high-speed processing, reliability and accuracy are very important and need to be better than humans.
Currently, most autonomous vehicles are using GPU (graphic processing chip) for their core AI processing. GPU is not as fast or cost-effective as a custom chip (ASIC). Ultimately, we need a dedicated AI autonomous processor. However, one of the biggest issues is power consumption. For above L3 to work flawlessly you need 100’s and 1000’s of watts to process real-time HD input from multiple cameras, radar, LiDAR, etc. That’s an enormous power requirement. It essentially means you need a dedicated battery for processing.
Autonomous Sensors
Looking back at accidents or issues in autonomous driving although in certain cases machine learning did not quite know how to react or reacted wrongly, in most cases the underlying factor has been driver negligence. Over 90% of car accidents are due to human errors and although human mistakes can be forgiven, machine errors are not. AI accuracy can be increased due to learning capabilities and algorithms used. Besides algorithms, infrastructure and government regulations are important to make autonomous driving possible. Above L3 (L3+, L4, and L5) scenarios are currently being evaluated by several top-tier companies in highway road conditions.
Cameras are typically used for vision processing, which is becoming aware of the surrounding environment for object detection, identification, segmentation, (lane) tracking, blind-spot monitoring, parking assist, traffic sign recognition, and color information. Cameras typically do not provide distance information. LiDAR is mostly used for 360-point detection with high accuracy and resolution. It can be used for traffic jams, AEB (automatic emergency brake), highway pilot, and more. LiDAR is very expensive and offers no color information. Radar is used for object detection with high-to-low resolution and is useful at long range, but cannot distinguish what the objects are. Ultrasonic is often used for parking assist, blind spots, ACC (automatic cruise control) with stop and go. Ultrasonic is useful for cruise control, collision avoidance, distance sensors, and weather resistance with low resolution. While cameras are important for the safe and effective operation of autonomous vehicles, LiDAR, radar, and ultrasonic provide more complete and reliable detection of the environment than camera sensors alone, particularly at night, foggy, or harsh weather conditions.
AI enables these sensors in three ways. First, sensing the surrounding environment is captured with cameras inside/outside, LiDAR, radar, and ultrasonic. Then perceiving through AI compute processing, algorithms, training, inferencing, and data/sensor fusion, which is used for data structuring, segmentation, object detection, and video understanding. Next is planning with context-awareness, path planning, and task prioritization. Lastly, is actuate/control which is steering, braking, acceleration and engine and transmission control.
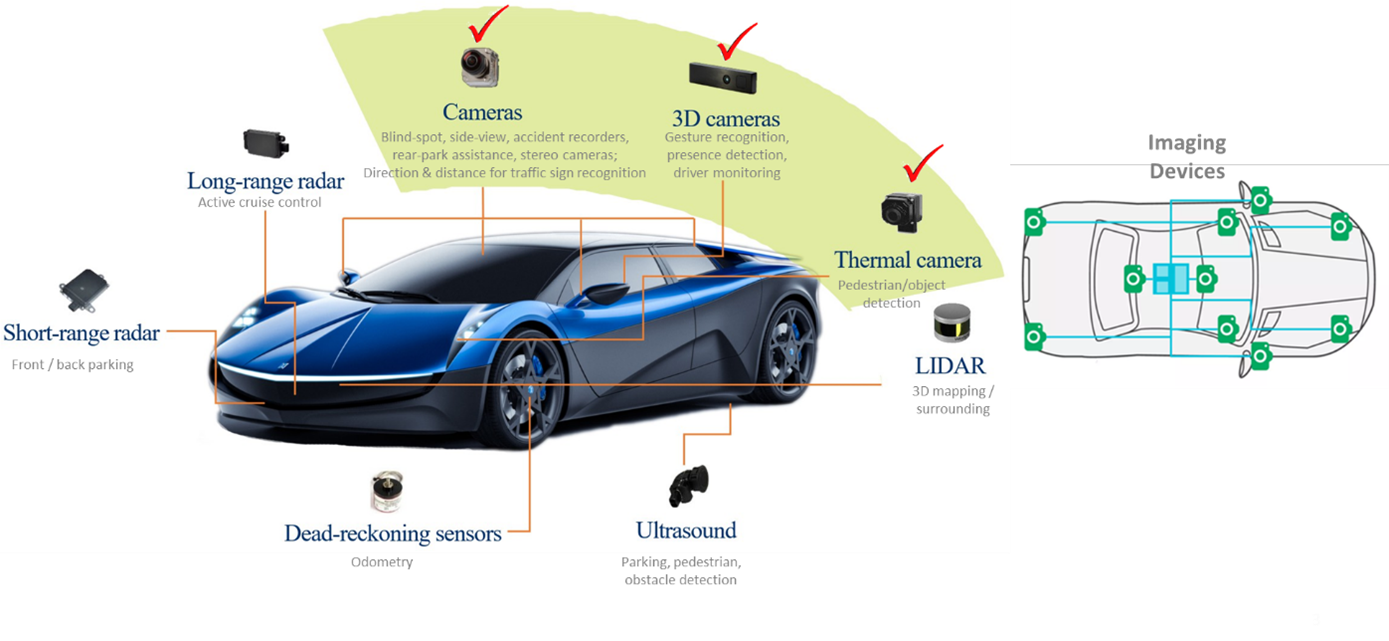
Figure 1 – Sensors (camera, LiDAR, Radar, Ultrasound) required for autonomous vehicle levels
The convergence of deep learning, edge computing, and the Internet of vehicles is driven by the recent advancements in AI automotive and vehicular communications. Another enabling technology for machine-oriented video processing and coding in visual data applications and industries is the emerging MPEG Video Coding for Machine (MPEG-VCM) standard.
Two specific technologies are investigated for VCM:
- Efficient compression of video/images
- The shared backbone of feature extraction
Powerful AI accelerators for inferencing at the edge, standard-based algorithms for video compression and analysis for machines (MPEG-VCM), and 5G connected vehicles (V2X) play a crucial role in enabling the full development of autonomous vehicles.
The 5G-V2X and emerging MPEG-VCM standards enable the industry to work towards harmonized international standards. The establishment of such harmonized regulations and international standards will be critical to global markets of future intelligent transportation and AI automotive industry.
There are a number of possible joint VCM-V2X architectures for the future autonomous vehicle (AV) industry. Depending on the requirements for the given AV infrastructure scenarios, we can have either centralized, distributed or hybrid VCM-V2X architectures as shown in Figure 2. Currently, most connected car automaker manufactures are experimenting with the centralized architecture with low-cost cameras. However, as the cameras become more intelligent, distributed, and hybrid architectures due to their scalability, flexibility, and resource sharing capabilities can become more attractive. The emerging MPEG-VCM standard also provides the capability of transporting the compressed extracted features rather than sending compressed video/images between vehicles.
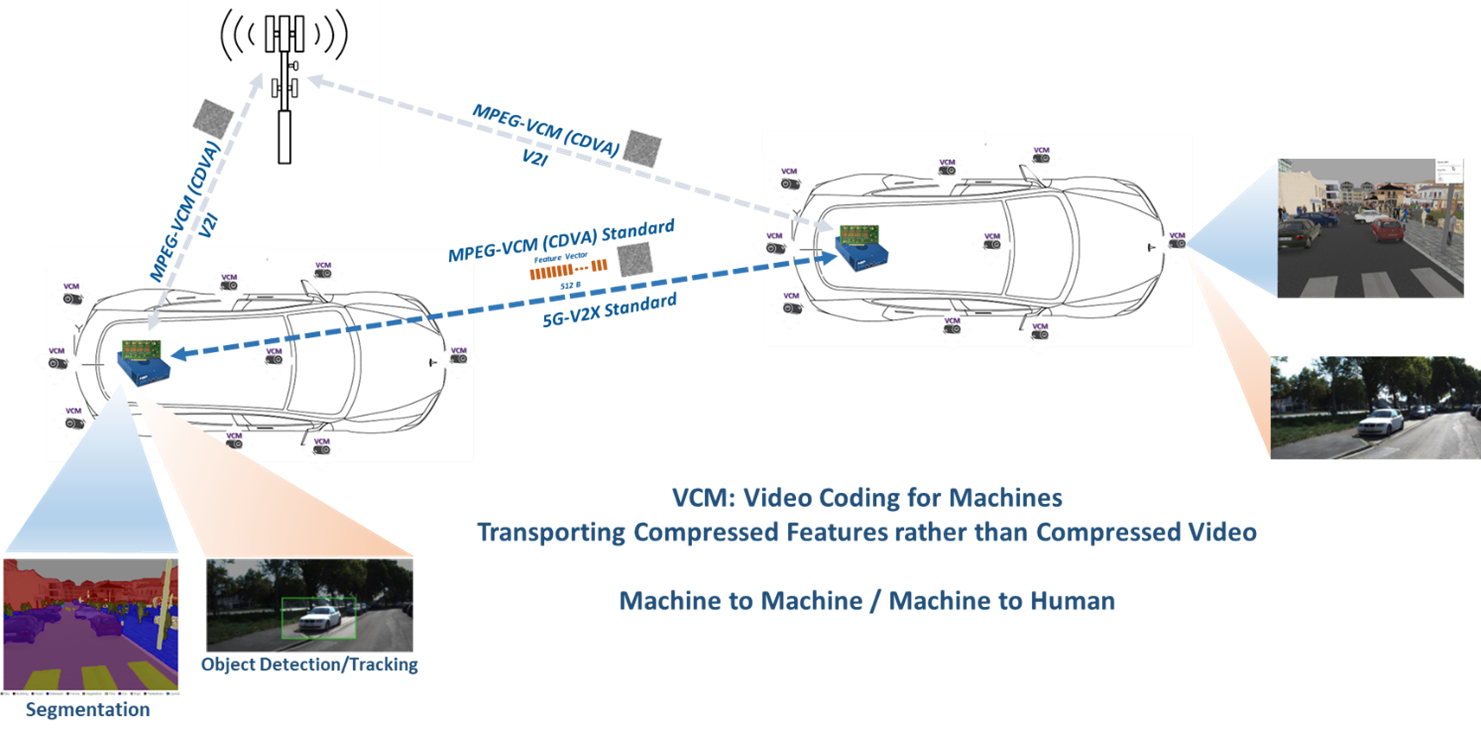
Figure 2 – Cooperative V2X-VCM: Internet of Vehicles (IoV)
Gyrfalcon Technology Inc. is at the forefront of these innovations by using the power of AI and deep learning to deliver a breakthrough solution for AI-powered cameras and autonomous vehicles — an unmatched performance, power efficiency, and scalability for accelerating AI inferencing at the device, edge, and cloud level.
The industry will require immense computing and processing power for all the information that AVs currently or in the future rely on with 5G. The convergence of 5G, edge computing, computer vision, and deep learning, and Video Coding for Machine (VCM) technologies will be key to fully autonomous vehicles. Standard and interoperable technologies such as V2X, emerging MPEG-VCM standard, powerful edge, and onboard compute inferencing accelerator chips enable low-latency, energy-efficient, low-cost, and safety benefits to the demanding requirements of the AI automotive industry.
___________________________________________
About Dr. Manouchehr Rafie
Dr. Manouchehr Rafie is Vice President of Advanced Technologies at Gyrfalcon Technology Inc. (GTI), which is the world’s leading developer of high-performance AI Accelerators that use low power, packaged in low-cost and small-sized chips. GTI drives the adoption of AI by bringing the power of cloud Artificial Intelligence to the edge and local devices with greater performance and efficiency.